2009/11/01
258. zenbakia
eu es fr en cat gl
Aparecerá un contenido traducido automáticamente. ¿Deseas continuar?
Un contenu traduit automatiquement apparaîtra. Voulez-vous continuer?
An automatically translated content item will be displayed. Do you want to continue?
Apareixerà un contingut traduït automàticament. Vols continuar?
Aparecerá un contido traducido automaticamente. ¿Desexas continuar?
Size matters: large collections of texts, necessary in language processing
Text created by automatic translator Elia and has not been subsequently revised by translators.
Elia Elhuyar
From the beginning of attempts to teach languages to machines intuitive and simplifying approaches have been used. Linguistic knowledge of linguists passed to structures that machines understood with the help of computer scientists, and through them language was treated. However, in recent years more and more techniques are based on large corpus and purely statistical methods.
Size matters: large collections of texts, necessary in language processing
01/11/2009 | Leturia Azkarate, Igor | Computer scientist and researcher
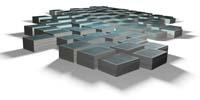
(Photo: Guillermo Roa)
Language processing exists almost since the creation of computers. The first programmable electronic machines created in the 40s of the last century, due to World War II, were mainly used to decipher messages and break codes, but, after the war, began to work a lot in language processing, especially in the field of machine translation.
In those beginnings, especially mathematicians, they used very simple techniques, influenced by the customs of cryptography: basically they intended to obtain machine translation through dictionaries and modifications of the word order. But they soon realized that languages were more than that, and that more complex language models had to be used. Thus, linguists were incorporated into the groups and applied the theories of Saussure and Chomsky. Since then, and over decades, in all areas of language processing (morphology, spelling correction, syntax, disambiguation of meanings...) an approach has predominated: the adaptation of knowledge based on the intuition of linguists to simple structures that can be treated by computers (rules, trees, graphs, programming languages...).
But these methods also have their limitations. On the one hand, the best linguists cannot take into account all the casuistry offered by a language; on the other hand, languages have a great complexity and richness to express themselves through simple structures. These limitations are even greater in conversational language. However, there was no other way; given the capacity of the machines of the time, this was the only way to speak with language. And with these techniques progress has been relatively slow for many years.
Arrival of corpus and statistics
However, in the last two decades, a more empirical approach is dominating language processing, based on the exploitation of large collections of texts and statistical methods. Rather than relying on intuitive knowledge, large real language samples, i.e. corpus, are used to take into account as many cases as possible of the language. Methods such as statistics or machine learning are used on them, with few linguistic techniques. Even in cases where language modeling is attempted through computable structures, the models are automatically extracted from the corpus. Therefore, working with statistical methods, in order for a machine to speak, it must have access to a huge collection of texts and resources to work with it.
This methodological change is mainly due to two factors. On the one hand, current computers, unlike previous ones, have the ability to handle huge amounts of data. On the other hand, there are more texts available in electronic format than ever, especially since the creation of the Internet.
Thus, corpus and statistical techniques are used in spell checkers (looking for contexts similar to the incorrect word in corpus), in machine translation (using translation memories or texts from multilingual websites to statistically obtain translations of words, syntagmas or phrases as large as possible), in sense disambiguation, in automatic terminology extraction, etc. And in general it can be said that the larger the corpus, the better results the systems get. For example, Google's Franz Joseph Och presented at the 2005 ACL (Association for Computational Linguistics) congress his statistical machine translation system, trained on a corpus of 200 billion words. And since then your system is the main reference in machine translation and the one that wins all contests. Something similar happens in other areas.
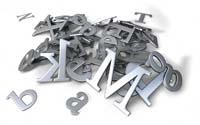
(Photo: iStockphoto.com/chieferu)
Future, hybridization
However, this methodology also has limitations. Some languages and tasks already use really gigantic corpus, and it can be said that they have already reached the top level, since they are very difficult to continue improving much more the results obtained. In other languages and areas there is no such large corpus, and with exclusively statistical methods such good results cannot be obtained.
Therefore, the recent tendency to improve statistical methods is to combine them with linguistic techniques and create hybrid methods. And in the future that will be the way forward in language processing. If we want machines to understand and deal with language shortly, and we want machines to speak, it will be necessary for mathematicians, computer scientists and linguists to go hand in hand.
Adam Kilgarriff: "Giant text-to-text databases can be collected relatively easily"
The use of corpus in the processing of language has been a revolution in recent years, and certainly the English Adam Kilgarriff has witnessed it. He has worked for years with corpus in English, and today he is a reference in the use of the internet as corpus. Sketch Engine (www.sketchengine.co.uk) is one of the founders of the tool that serves to work in this line. He participated in the 2009 SEPLN congress on language processing organized by the IXA group of the UPV in Donostia.
What are the main difficulties for a machine to speak?
There are many. Man knows many things. Since we were born we are learning, seeing, perceiving... we have a lot of wisdom in our heads and we know what the relationship between ideas is. The 50-year research is not enough for artificial intelligence to do the same. We have all the data in our heads. Hence the greatest difficulty that speaking machines have: we have not yet succeeded in making a lot of materials useful for the computer.
On the other hand, we have many problems related to language. There are many ways to say anything, and for computers it is very difficult to understand that two sentences express the same idea. You won't understand that the phrases "This place is wonderful" and "Here's a beautiful beach" basically express the same idea. Otherwise, a single phrase can have more than one meaning. The phrase "I have seen a mouse" has different meaning in the Miramar Palace or in a biology laboratory.
These are the main general problems (but there are many other small ones).
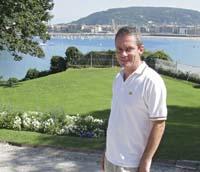
(Photo: Guillermo Roa)
Is it necessary to use artificial intelligence in language processing?
Machine learning is being used for more and more things in language processing. But artificial intelligence is not only one thing, but many strategies have been developed in different areas. The approach that interests me for the treatment of the language is to find patterns from many data. A child does, looks for patterns in sounds, meanings, grammar, etc. and it is what creates the lexicon of the child. It is our work. For example, we start from a word and with it we look for words that appear in the same context.
Machine learning, for example, allows you to search for patterns and build knowledge by computer. It is, therefore, a way of dealing with one of the main problems of language processing, that is, of solving a case in which a single word has more than one meaning. This is possible if we use large corpus.
The best corpus is the Internet?
It depends on the objective. In many of my jobs, the more data I use, the better it works. But the network also poses some difficulties. There is a lot of spam. Therefore, the best strategy to manage this data is the one used by Google and Yahoo: collect many websites and search only the text to work with less information (in a gigabyte few videos enter, but there is a lot of text). In this way, giant databases can be collected relatively easily to text. Today, the largest English has 5.5 billion words. And of this type you can find many patterns.
The problem is that the language in which a machine will talk should not be, for example, the same style that scientists write in the articles. It should be the language in which we speak. Therefore, it does not serve for this purpose a large corpus of texts written in articles or newspapers. You need a large corpus based on conversation, based on chats. But it is difficult to collect these texts and confidentiality makes it even more difficult. For our research we collect texts from blogs where writing has less formality.
Leturia Azkarate, Igor
Services
258
2009
Results
020
Robotics
Article
Services